-
Hasnain Haider
- Reading Time: 30 Min
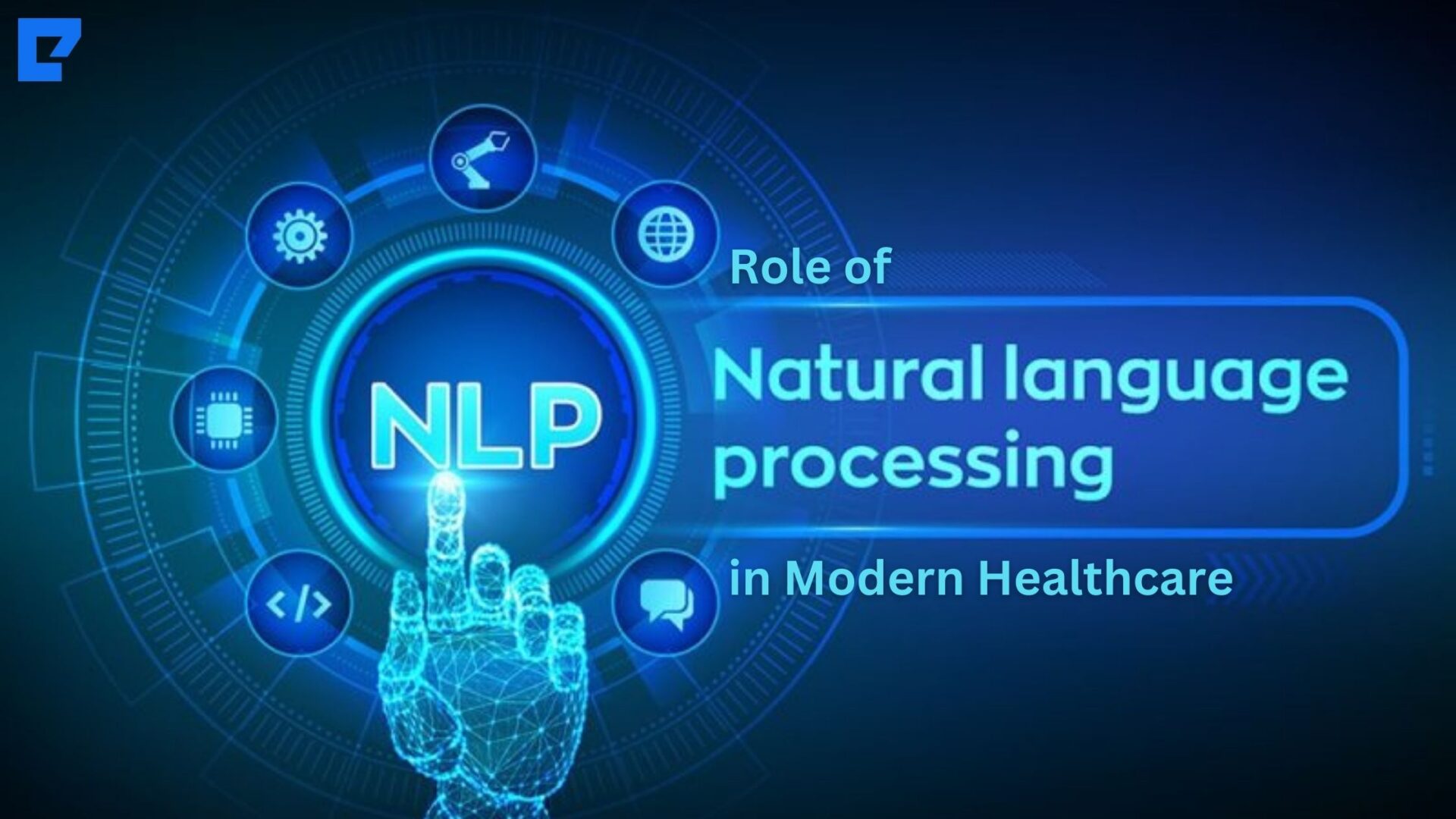
1. Introduction
Natural Language Processing (NLP) has rapidly become a transformative force in healthcare, reshaping how professionals interact with data and make decisions. As a subset of artificial intelligence (AI), NLP enables computers to understand, interpret, and respond to human language. In an industry where vast amounts of unstructured data—clinical notes, patient records, research publications—are generated daily, this capability is essential.
NLP’s role in modern healthcare extends far beyond simple automation. It allows for the seamless extraction of critical information from unstructured data, turning it into actionable insights that can enhance patient care, streamline operations, and improve overall efficiency. From automating clinical documentation to analyzing patient feedback, NLP tools are proving invaluable in addressing the time-intensive and error-prone tasks that healthcare professionals face.
Moreover, as healthcare systems integrate with increasingly advanced IT solutions like Electronic Health Records (EHR) and predictive analytics, NLP serves as the connective tissue that helps unify these technologies. It ensures that critical insights are accessible at the right time, enabling more informed decision-making at every stage of the care process.
In this article, we will explore how NLP is revolutionizing healthcare by examining its current applications, the challenges it faces, and the skills needed to develop effective solutions.
2. Current Applications of NLP in Healthcare
Natural Language Processing (NLP) has become an essential tool in healthcare, addressing the challenges posed by the vast amounts of unstructured data generated in clinical environments. By leveraging NLP, healthcare organizations can enhance patient care, streamline administrative processes, and improve data accuracy and accessibility. Below, we explore some of the most impactful applications of NLP in modern healthcare.
2.1 Clinical Documentation and EHR Automation
One of the most significant applications of NLP is in clinical documentation and automating Electronic Health Records (EHR). Healthcare professionals spend a considerable amount of time transcribing patient notes, which often leads to inefficiencies and errors. NLP systems can automatically convert unstructured clinical notes—whether handwritten or spoken—into structured, searchable data within the EHR. This process not only reduces the administrative burden on doctors and nurses but also enhances the accuracy of medical records.
For example, NLP tools can extract key information such as diagnoses, medications, and procedures from physician notes and populate the corresponding fields in the EHR. This ensures that critical patient information is always up-to-date and easily accessible, contributing to better care decisions and reducing the risk of human error.
2.2 Clinical Decision Support Systems
NLP plays a crucial role in Clinical Decision Support Systems (CDSS), where its ability to analyze large volumes of data quickly can provide invaluable insights for healthcare providers. These systems integrate medical literature, patient records, and diagnostic data to assist clinicians in making informed decisions about diagnosis and treatment plans. By processing unstructured data from medical journals, case studies, and research papers, NLP tools can surface relevant findings that a clinician might otherwise overlook due to the sheer volume of information.
For instance, a CDSS powered by NLP could analyze a patient’s medical history alongside the latest research on similar cases, offering suggestions for treatment based on data-driven insights. This capability not only improves the accuracy of diagnoses but also accelerates the time it takes to arrive at critical decisions, especially in complex cases.
2.3 Enhancing Patient Interaction and Engagement
Patient engagement is another area where NLP has made significant inroads. Healthcare providers are increasingly using NLP-powered chatbots and virtual assistants to enhance communication with patients. These tools can handle a wide range of tasks, from answering basic health queries to managing appointment scheduling and medication reminders. By automating routine interactions, healthcare providers can focus on more complex patient care, while patients enjoy a more streamlined and responsive service.
Additionally, NLP is used to analyze patient feedback from surveys, reviews, and social media, offering insights into patient satisfaction and identifying areas for improvement. Sentiment analysis, a technique within NLP, helps healthcare organizations gauge patient emotions and sentiments about their care experience, allowing them to make data-driven decisions to enhance patient satisfaction and service quality.
3. Integration of NLP with Other IT Solutions
The true power of Natural Language Processing (NLP) in healthcare lies in its ability to integrate with other IT solutions, creating a seamless flow of information across various healthcare systems. This integration enhances the capabilities of Electronic Health Records (EHR), predictive analytics, and AI-driven diagnostics, enabling healthcare providers to deliver more precise, timely, and personalized care. In this section, we explore how NLP works alongside these IT solutions to revolutionize healthcare operations.
3.1 NLP in Predictive Analytics for Better Patient Outcomes
Predictive analytics, driven by machine learning algorithms, plays a crucial role in modern healthcare by analyzing historical data to predict future patient outcomes. When integrated with NLP, predictive analytics becomes even more powerful. Healthcare organizations generate massive amounts of unstructured data—clinical notes, patient histories, and medical literature—that can be difficult to analyze with traditional tools. NLP bridges this gap by transforming unstructured data into structured insights, making it easier to incorporate into predictive models.
For example, NLP can analyze past clinical notes to detect subtle patterns or early symptoms that may indicate the likelihood of a patient developing a chronic condition, such as heart disease or diabetes. By identifying these patterns early, healthcare providers can intervene sooner, potentially preventing severe health issues and improving long-term patient outcomes. Furthermore, predictive models powered by NLP can help healthcare providers identify high-risk patients and tailor interventions to their specific needs, driving more personalized care.
3.2 Merging NLP with Machine Learning and AI Diagnostics
NLP also plays a critical role in enhancing AI-driven diagnostic tools. As the volume of healthcare data grows, machine learning algorithms require accurate and structured data to make reliable predictions and diagnoses. NLP ensures that unstructured data, such as doctor’s notes, lab reports, and imaging study results, are accurately processed and fed into AI models, enabling these systems to deliver more accurate diagnostic results.
For instance, an AI diagnostic tool could use NLP to extract key details from a patient’s medical history and compare them to patterns found in similar cases. This could assist healthcare providers in diagnosing complex diseases, identifying rare conditions, or even proposing potential treatment plans based on the latest medical research. The integration of NLP with AI diagnostics helps improve the speed and accuracy of diagnosis, ultimately leading to better patient care and outcomes.
3.3 Streamlining Interoperability in Healthcare IT Systems
Interoperability—the ability for different healthcare systems to communicate and share data—is a critical challenge in the industry. Many healthcare organizations rely on multiple IT solutions, including EHR systems, radiology information systems, and laboratory management systems, that often operate in silos. NLP helps break down these silos by enabling different systems to understand and communicate unstructured data.
For example, NLP can facilitate the seamless exchange of information between a hospital’s EHR system and a specialized diagnostic system, ensuring that all relevant patient data is accessible across platforms. This interoperability not only improves workflow efficiency but also ensures that healthcare professionals have a complete view of the patient’s medical history, enabling more accurate and informed clinical decisions.
3.4 Enhancing Communication with NLP-Powered Virtual Assistants
Another key integration point for NLP is in virtual assistant technology. These AI-powered assistants can integrate with EHR systems, predictive analytics, and diagnostic tools to streamline communication between healthcare professionals and patients. For example, a virtual assistant can help clinicians retrieve important patient information from the EHR by using simple voice commands, reducing the need for manual searches and allowing them to focus on patient care.
In patient-facing applications, virtual assistants can answer routine health questions, schedule appointments, and provide medication reminders. This integration not only improves patient engagement but also helps healthcare providers manage their workload more efficiently by automating routine tasks.
4. Benefits of NLP in Healthcare
Natural Language Processing (NLP) is driving a new wave of efficiency, accuracy, and personalization in healthcare. By enabling the analysis and utilization of unstructured data, such as clinical notes, medical records, and research publications, NLP offers a wide array of benefits that enhance both the operational and clinical aspects of healthcare. In this section, we explore the key advantages NLP brings to the healthcare industry, demonstrating how it is transforming patient care, administrative workflows, and compliance practices.
4.1 Enhancing Accuracy and Speed in Medical Coding
One of the most impactful benefits of NLP in healthcare is its ability to streamline medical coding, an essential process for accurate billing and insurance claims. Traditionally, healthcare providers manually convert clinical notes into medical codes (such as ICD-10), a time-consuming and error-prone process. NLP automates this task by extracting relevant terms from clinical documentation and matching them to the appropriate medical codes.
By enhancing the accuracy and speed of coding, NLP reduces the likelihood of billing errors, improves claim approval rates, and shortens the revenue cycle. For healthcare organizations, this translates into reduced administrative costs and more efficient financial operations. Additionally, accurate coding ensures that patient care is properly documented, supporting better outcomes and more reliable data for analysis.
4.2 Reducing Administrative Burden on Healthcare Professionals
Healthcare professionals often face overwhelming administrative workloads, from updating patient records to transcribing notes and managing appointments. NLP alleviates much of this burden by automating repetitive and time-intensive tasks, allowing clinicians to focus more on patient care. For instance, NLP tools can automatically convert spoken or handwritten notes into structured formats within Electronic Health Records (EHR), eliminating the need for manual data entry.
This reduction in administrative work not only boosts productivity but also improves the quality of care. By freeing up more time, healthcare professionals can spend more time on direct patient interaction, diagnostics, and treatment planning. Moreover, it minimizes burnout, which is a growing concern among healthcare workers, especially in high-stress environments.
4.3 Improving Clinical Decision-Making and Patient Outcomes
NLP significantly enhances clinical decision-making by providing healthcare professionals with access to relevant and timely information. Through NLP-powered Clinical Decision Support Systems (CDSS), clinicians can quickly retrieve critical insights from a patient’s history, research databases, or medical literature to make evidence-based decisions. This is particularly valuable in complex cases where the sheer volume of available information can be overwhelming.
For example, NLP can analyze patient records to identify trends or risk factors that might not be immediately obvious, such as early warning signs for chronic conditions. By uncovering these insights, healthcare providers can intervene earlier, improving patient outcomes through preventive care. Furthermore, NLP-driven decision support can help clinicians choose the most effective treatments, tailored to each patient’s unique medical history.
4.4 Data Anonymization and Privacy Compliance
In a healthcare industry governed by stringent privacy regulations, such as HIPAA in the United States, safeguarding patient data is of paramount importance. NLP plays a critical role in ensuring data privacy by enabling efficient data anonymization. When processing sensitive patient information, NLP systems can automatically remove or replace personally identifiable information (PII) with semantic tags, ensuring that the data remains compliant with privacy regulations.
This capability is especially beneficial for healthcare organizations that need to share patient data for research, analytics, or collaboration with other institutions. By anonymizing data, NLP facilitates safe data sharing without compromising patient privacy. This not only enhances regulatory compliance but also opens up opportunities for collaborative research and innovation.
4.5 Enabling Personalized Patient Communication
Effective communication between healthcare providers and patients is crucial for delivering high-quality care. NLP enhances this communication by simplifying complex medical language into more accessible terms, ensuring that patients fully understand their diagnoses, treatment plans, and medications. Additionally, NLP-powered tools, such as virtual assistants and chatbots, can engage patients by answering their questions, scheduling appointments, and providing reminders for medications or follow-up visits.
By making communication more personalized and responsive, NLP improves patient engagement, adherence to treatment plans, and overall satisfaction. This is especially valuable in chronic disease management, where regular patient interaction and adherence to care plans are critical to achieving positive outcomes.
The benefits of NLP in healthcare are far-reaching, touching on everything from operational efficiency to patient engagement and clinical accuracy. As healthcare systems continue to evolve, the role of NLP will expand, driving even greater improvements in how care is delivered, documented, and managed. In the next section, we will discuss the challenges that healthcare organizations face when implementing NLP solutions, as well as the skills required to overcome these obstacles.
5. Challenges of Implementing NLP in Healthcare
While Natural Language Processing (NLP) has the potential to transform the healthcare industry, its implementation comes with a set of significant challenges. These challenges, ranging from the complexity of medical language to concerns over data privacy, can hinder the widespread adoption of NLP solutions. In this section, we explore the key obstacles that healthcare organizations face when integrating NLP into their operations and how these challenges can be addressed.
5.1 Overcoming the Complexity of Medical Terminology
One of the biggest hurdles in implementing NLP in healthcare is the complexity and specificity of medical language. Medical terminology is often highly technical, filled with abbreviations, acronyms, and jargon that can vary between specialties and regions. Additionally, clinicians may use different terms to describe the same condition or procedure, which makes it difficult for NLP systems to accurately interpret and extract meaning from unstructured data.
For NLP to be effective in healthcare, it must be trained on large and diverse datasets that account for this variability in medical language. However, acquiring such datasets can be challenging due to the sensitive nature of healthcare data and the cost involved in curating them. Moreover, NLP models need continuous updates to keep up with new medical discoveries, evolving terminology, and treatment protocols. Addressing these linguistic complexities requires collaboration between healthcare professionals, data scientists, and technology providers to ensure that NLP systems are properly tailored to the nuances of the medical field.
5.2 Data Security and Privacy Concerns
Healthcare data is among the most sensitive information, and protecting patient privacy is paramount in any technology deployment. With the implementation of NLP, there are increased concerns over data security, especially as these systems process vast amounts of personal health information (PHI). Ensuring compliance with regulations like the Health Insurance Portability and Accountability Act (HIPAA) in the U.S. or the General Data Protection Regulation (GDPR) in Europe is critical when handling patient data.
NLP tools must incorporate robust security features, such as encryption and anonymization, to safeguard PHI from unauthorized access or breaches. Additionally, healthcare organizations must ensure that NLP solutions comply with regulatory standards by conducting regular audits and assessments. Despite the advances in data anonymization through NLP, maintaining patient privacy remains a challenge, particularly when sharing data across institutions for research or analytics purposes. The risk of de-anonymization—where re-identification of patient data could occur—remains a pressing concern.
5.3 Integration Challenges with Existing Systems
Another major obstacle to NLP adoption in healthcare is the challenge of integrating these systems with existing IT infrastructure, particularly Electronic Health Records (EHR). Many healthcare organizations still rely on legacy systems that were not designed to accommodate advanced technologies like NLP. This lack of interoperability between new NLP solutions and existing healthcare IT systems can lead to costly and time-consuming integration processes.
Incompatible data formats, inconsistent workflows, and fragmented data silos further complicate this integration. Healthcare providers often face difficulties in merging structured data from EHR systems with the unstructured data processed by NLP tools. Moreover, the diverse range of EHR vendors, each with their own proprietary systems, adds another layer of complexity. For NLP to be fully effective, it must seamlessly integrate with these existing systems to enable real-time access to patient information and clinical insights.
To overcome these challenges, healthcare organizations need to invest in IT infrastructure modernization and prioritize interoperability when selecting NLP tools. Collaboration between NLP vendors, EHR providers, and healthcare institutions is critical to developing standardized solutions that work across multiple platforms.
5.4 Ensuring Accuracy and Reducing Bias
NLP models, like all machine learning systems, are only as good as the data they are trained on. In healthcare, ensuring the accuracy of NLP predictions and minimizing bias is particularly important, as errors in diagnosis, treatment recommendations, or patient sentiment analysis can have serious consequences. If an NLP model is trained on biased or incomplete datasets, it could reinforce existing disparities in healthcare, such as underdiagnosis of certain conditions in minority populations.
To mitigate this risk, NLP models need to be trained on diverse datasets that reflect the wide range of patient demographics and medical conditions. Continuous testing and validation of these models are essential to ensure their accuracy and fairness in real-world applications. Additionally, human oversight is necessary to identify and correct any biases that may arise during the deployment of NLP systems.
Implementing NLP in healthcare presents numerous challenges, from handling the intricacies of medical language to ensuring data privacy and system integration. However, addressing these obstacles is critical for realizing the full potential of NLP in transforming healthcare. In the next section, we will discuss the skills and expertise required for IT professionals to develop and manage effective NLP solutions in this complex industry.
6. Skills Needed for Developing NLP Applications in Healthcare
Developing effective Natural Language Processing (NLP) applications in healthcare requires a unique blend of technical expertise and domain-specific knowledge. The complexity of medical language, the sensitivity of healthcare data, and the need for accurate, unbiased outcomes make it essential for developers to possess a robust skill set. In this section, we’ll explore the key skills IT professionals must cultivate to create impactful NLP solutions in healthcare.
6.1 Expertise in NLP and AI Technologies
At the core of building NLP applications is a deep understanding of the algorithms and models that power these systems. IT professionals must be proficient in a variety of machine learning (ML) techniques, particularly those related to text processing and natural language understanding. Familiarity with models like recurrent neural networks (RNNs), transformers (such as BERT and GPT), and deep learning frameworks (such as TensorFlow or PyTorch) is essential. These models enable machines to recognize, interpret, and generate human language, which is foundational for NLP applications in healthcare.
Developers must also understand language-specific tools, such as tokenization, named entity recognition (NER), and sentiment analysis, as these techniques are often used to process and extract valuable information from clinical notes, patient records, and other unstructured data sources. Additionally, a firm grasp of data pre-processing techniques, including the cleaning and normalization of text, is crucial for ensuring that NLP models can accurately interpret medical terminology and context.
6.2 Knowledge of Healthcare Regulations and Data Standards
In healthcare, compliance with regulatory frameworks like HIPAA (in the U.S.) or GDPR (in Europe) is non-negotiable. IT professionals working on NLP applications must have a strong understanding of these regulations to ensure that their solutions meet data privacy and security requirements. This includes familiarity with anonymization techniques, data encryption, and secure data storage protocols, all of which are critical for protecting sensitive patient information.
Additionally, developers must be well-versed in healthcare-specific data standards, such as ICD-10 for medical coding or HL7 and FHIR for electronic health record (EHR) interoperability. These standards ensure that the NLP applications can effectively communicate with other healthcare systems, enabling seamless integration and data sharing. Understanding the nuances of these standards is key to building systems that can accurately interpret and exchange data between different healthcare IT solutions.
6.3 Proficiency in Medical Terminology and Clinical Workflows
One of the unique challenges of developing NLP applications for healthcare is the complexity of medical language and clinical workflows. IT professionals need to have a working knowledge of medical terminology, including common abbreviations, acronyms, and the contextual usage of specific terms across different medical specialties. This is especially important for tasks such as entity recognition, where NLP models must accurately identify conditions, medications, and procedures from clinical notes.
In addition to medical terminology, developers must understand the workflows and processes within healthcare settings. This includes knowing how data is generated, recorded, and used by healthcare professionals. For example, understanding how a clinician documents patient encounters or how laboratory results are incorporated into a patient’s record can help developers design NLP systems that fit seamlessly into existing workflows, enhancing efficiency rather than disrupting it.
6.4 Data Science and Statistical Analysis
A strong foundation in data science is indispensable for developing NLP applications, particularly when working with healthcare data. IT professionals must be able to design, train, and evaluate machine learning models, requiring skills in statistical analysis, data visualization, and model validation. These skills are essential for interpreting the vast amounts of data generated by healthcare systems and ensuring that NLP models provide accurate, reliable insights.
In healthcare, where the stakes are high, it is also critical to continuously monitor and refine NLP models to ensure their effectiveness over time. This requires expertise in A/B testing, model performance metrics, and ongoing model updates based on new data. IT professionals need to be comfortable working with both structured and unstructured data, as NLP applications in healthcare often involve the integration of clinical notes with other data sources like lab results, imaging studies, and patient histories.
6.5 Collaboration with Healthcare Professionals
Finally, developing successful NLP applications for healthcare requires close collaboration with healthcare professionals. IT developers must be able to communicate effectively with clinicians, administrators, and other stakeholders to understand their needs and ensure that NLP tools are solving real-world problems. This collaboration is essential for refining the design and functionality of NLP solutions, ensuring that they align with clinical goals and contribute to improved patient care.
By working directly with healthcare providers, IT professionals can gain insights into the practical challenges faced in medical environments, such as time constraints, documentation burdens, and decision-making processes. This collaboration ensures that the NLP applications they develop are both user-friendly and highly effective in supporting clinical decision-making and operational workflows.
7. Conclusion: The Future of NLP in Healthcare
As the healthcare industry continues to evolve, Natural Language Processing (NLP) is poised to play an even more transformative role in how patient care is delivered and how healthcare systems operate. The ability of NLP to process, analyze, and derive meaningful insights from vast amounts of unstructured data opens up new possibilities for improving clinical outcomes, reducing administrative burdens, and enhancing patient engagement. However, the future of NLP in healthcare will depend on how well the industry can address the challenges associated with implementation, security, and interoperability.
One of the most promising aspects of NLP’s future is its potential to drive more personalized and predictive healthcare. As NLP technologies become more sophisticated, they will enable healthcare providers to deliver individualized treatment plans based on a patient’s specific medical history, risk factors, and current conditions. Predictive analytics, fueled by NLP’s ability to process unstructured clinical notes and other data sources, will allow healthcare providers to anticipate health issues before they become critical. This shift from reactive to proactive care will be a significant milestone in improving patient outcomes and reducing the overall cost of care.
Moreover, NLP will likely play a critical role in making healthcare more accessible. NLP-powered virtual assistants and chatbots are already being used to handle routine patient inquiries and administrative tasks, but future iterations of these tools could provide even more advanced support. For instance, NLP could be integrated into telemedicine platforms, enabling real-time analysis of conversations between patients and doctors, flagging critical issues, and suggesting follow-up questions or treatments. This would not only improve the efficiency of virtual consultations but also ensure that important details are not overlooked, especially when managing chronic conditions or monitoring high-risk patients.
Another key area where NLP will continue to grow is in research and clinical trials. The ability to sift through massive amounts of medical literature and clinical trial data in real-time allows researchers to identify emerging trends, new treatment options, and potential drug interactions more quickly. As more healthcare organizations adopt NLP to enhance their research capabilities, the speed at which new treatments and therapies are developed could increase, leading to faster innovation in the medical field.
However, the future of NLP in healthcare also hinges on overcoming the current obstacles. Ensuring data security and patient privacy will remain critical as healthcare organizations use NLP to process sensitive information. As NLP technologies become more advanced, developers must continue to prioritize privacy by building in robust encryption, data anonymization, and compliance with regulatory standards like HIPAA and GDPR. Additionally, the challenge of integrating NLP with existing healthcare systems, particularly legacy Electronic Health Records (EHR), must be addressed through better interoperability solutions that enable seamless communication across platforms.
The skills needed to implement and maintain NLP systems in healthcare will also evolve. IT professionals will need to stay updated on the latest NLP techniques and tools while also deepening their understanding of healthcare regulations and workflows. Collaboration between data scientists, healthcare providers, and regulatory experts will be crucial to developing and deploying NLP solutions that meet both the technical and clinical demands of the industry.
In summary, the future of NLP in healthcare holds immense potential, with the capability to improve patient care, streamline operations, and accelerate medical research. As the industry addresses current challenges and invests in advancing NLP technologies, healthcare providers and patients alike stand to benefit from more efficient, effective, and personalized care. The road ahead for NLP in healthcare is filled with opportunities, and those organizations that embrace it early will be better positioned to lead in this new era of healthcare innovation.